- UPS uses an AI-powered GPS tool called ORION (On-Road Integrated Optimization and Navigation) to create the most efficient routes for its fleet, making changes in real time to account for road conditions and other factors. By optimizing delivery efficiency, the company estimates it saves $50 million a year.
- Clothing retailer Gap Inc. is using AI-assisted mechanical arms to help sort clothing orders.
- Connecticut-based XPO Logistics Inc. has begun roll-out of 5,000 intelligent robots throughout its logistics sites in North America and Europe. The robots are used to bring mobile storage racks full of products to workers who fill customer orders.
AI is now being used in every aspect of managing supply chain and manufacturing operations, says Chris Noyes, a senior manager with Deloitte Consulting LLP. Product development is using natural language processing on social media data to understand customer feedback. Logistics managers are using advanced optimization algorithms to find better network configurations. Risk managers are using web crawlers and predictive algorithms to surface concerns. Procurement is using machine learning to classify (procurement) spend, providing a better data foundation for spend analysis.
In manufacturing, smart factories are embracing “Internet of Things” platforms with machine learning functionality to improve operations. These platforms provide real-time visibility across every machine in the plant, Noyes notes. They record the decisions personnel have made, creating a data foundation that can be used to develop an intelligent agent. Predictive models identify anomalous behavior and guide maintenance activities.
Companies have been using AI to forecast future activity for some time, says Josh Nelson, a principal and supply chain consultant with The Hackett Group. “What has changed is that companies have access to more indicators and more structured data to understand what is happening, and more algorithms to better predict what is going to happen.” They are using it to understand what is happening in major markets, so they can respond by changing production schedules and moving inventory to where it is needed to meet demand, Nelson explains.
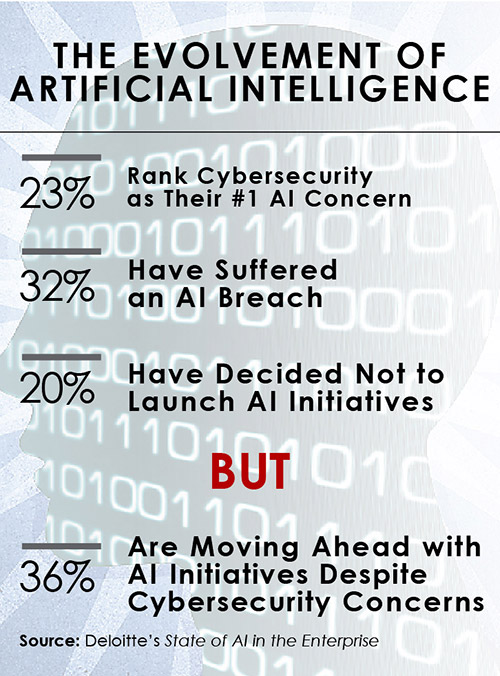
Companies are also investing heavily in material management technology. Most of the use of autonomous vehicles is happening within the controlled environment of a warehouse or similar building. With the right software, autonomous vehicles can “work wonders in that type of environment. Within four walls, there are a lot of options out there,” Nelson says. Using driverless vehicles in the “outside” environment is very appealing to employers, due to driver shortages in the transportation industry. But that use is still in the emerging technology-prototype stages.
One of the more immediate opportunities is the idea of having a remotely controlled drone system, which would be initially used within the confines of a city. “There could be a plant with a warehouse down the road that shuttles material back and forth. Developing software that will work in an external environment is much more complicated because more variables are involved than in a controlled environment,” Nelson notes. “And, with longer distances, there are regulatory hurdles.”
Another major category getting close to application is robotic process automation (RPA), Nelson adds. “A number of companies have moved beyond the pilot stage and are looking to deploy it more broadly in areas like accounts payable. Machines would process and validate automated payments. That depends on having standardized processes in place. Then, over time, your technology ‘learns’, and you can enhance the robotic/automation elements. We’re very close to that,” he says.
Companies have been using AI to forecast future activity for some time. What has changed is that companies have access to more indicators and more structured data to understand what is happening, and more algorithms to better predict what is going to happen. Josh Nelson, principal and supply chain consultant, The Hackett Group The more dynamic and nonstandardized an environment is, the more difficult it is to factor in all of the variables, Nelson points out. “Things that are more standard and contained lend themselves to being opportunities in the short term. With unknown factors (city or highway), a lot more has to be modeled and validated before you can roll it out in a big way.”
Another technology with great potential is blockchain, Nelson believes. A blockchain is a growing list of records, which are connected using cryptography. Used as a distributed ledger to record transactions, the encryption is employed to prevent the data from being modified by a hacker. “We’re seeing a lot of discussion about block chain in supply chain management. We’re going to see companies doing more pilots and proof-of-concept work — uses like traceability” in pharmaceutical inventories, for example.
The State of AI
Obviously, cybersecurity is a major concern in using AI. In Deloitte’s recent State of AI in the Enterprise survey, cybersecurity vulnerabilities ranked as a concern for half of respondents, with almost a quarter — 23 percent — ranking it as the number-one AI/cognitive technology risk, according to Nitin Mittal, principal and Analytics & Cognitive Offering leader with Deloitte. Thirty percent of the respondents have slowed an AI initiative to address cybersecurity concerns, and one in five has decided not to launch AI initiatives.
This apprehension is attributed to the potential of cyber-related liabilities surfacing for certain AI technologies, Mittal says. For example, some machine-learning models have difficulty detecting adversarial input or data that is constructed specifically to deceive the model. Recent incidents where AI was used to create fake photos and videos of celebrities and politicians — and given the prominence of AI-based image recognition — shows that this could become a big area of risk in the future. Worst-case scenarios, like autonomous vehicles getting hacked, also have life-threatening ramifications.
Although 32 percent of the Deloitte survey respondents have suffered an AI-related breach within the last two years, 36 percent said they are moving ahead with AI initiatives despite cybersecurity concerns. “While no cybersecurity effort will prevent every attack, enterprises should improve their defenses by incorporating security from the beginning of the process and making it a higher priority,” Mittal advises.
Another major category getting close to application is robotic process automation (RPA). A number of companies have moved beyond the pilot stage and are looking to deploy it more broadly in areas like accounts payable. Machines would process and validate automated payments. That depends on having standardized processes in place. Then, over time, your technology ‘learns’, and you can enhance the robotic/automation elements. We’re very close to that. Josh Nelson, principal and supply chain consultant, The Hackett Group For some companies, another challenge to implementing artificial intelligence in supply chain management is a tech skills gap. In Deloitte’s survey, 69 percent of respondents report that they are facing a “moderate, major or extreme” skills gap. Deloitte’s research suggests that the biggest skills currently needed are AI software developers (28 percent) and data scientists (24 percent) due to the need for innovation of new AI algorithms and systems.
Data also remains a top challenge when implementing AI initiatives, Mittal notes. Thirty-nine percent of respondents in the survey ranked data issues such as accessing/integrating data and privacy in their top three concerns, and 16 percent ranked them as number one. There are several reasons for this such as integrating siloed data (especially within organizations that have experienced acquisitions), analysis of unstructured data, privacy, and more. “All of this — while ensuring security — can be time-consuming, costly, and complex,” notes Mittal.
Another factor is trust, Mittal says. “Behavioral change remains an issue as humans and machines continue to learn how to work together. For example, an organization built a machine-learning system to support a sales team by predicting what prospects were likely to convert and which customers would churn. Though the system worked, the sales team was not prepared to accept its recommendations because they didn’t understand or trust the results that it produced. By bringing users of the systems into the design process of the new systems, they can experience first-hand how the technology works and how the insights are formed. In turn, the users who contributed to the development of the system will adopt it because they understand — and trust — the process.”
An Ongoing Process
AI, which originated with big tech companies, was then adopted by large companies in other industries. Mid-sized companies are now making the investment as methodologies have been refined and tools are more standard,” says Chris Noyes of Deloitte. “For example, one mid-size company we work with has leapfrogged from standard maintenance to cutting-edge machine learning-driven predictive maintenance. The biggest challenge for these companies will be attracting talent that thrives in the digital age, and they will need to partner with service providers to fill the gaps.”
Hackett Group’s Nelson says the roll out of these technologies will continue to happen on an incremental basis, over the next decade. “It’s not going to be an overnight ‘red to green’ process,” he concludes.